Image Recognition Using Artificial Intelligence IEEE Conference Publication
Automatic image recognition: with AI, machines learn how to see
This helps save a significant amount of time and resources that would be required to moderate content manually. AI-based image recognition can be used to detect fraud by analyzing images and video to identify suspicious or fraudulent activity. AI-based image recognition can be used to detect fraud in various fields such as finance, insurance, retail, and government.
Visual Search is a new AI-driven technology that allows the user to perform an online search using real-world images as text replacements. The use of AI for image recognition is revolutionizing all industries, from retail and security to logistics and marketing. In this section we will look at the main applications of automatic image recognition.
Pre-processing of the image data
Each feature produces a filtered image with high scores and low scores when scanning through the original image. For example, the red box found four areas in the original image that show a perfect match with the feature, so scores are high for those four areas. The act of trying every possible match by scanning through the original image is called convolution. The filtered images are stacked together to become the convolution layer.
Facial-recognition ban gets lawmakers’ backing in AI Act vote – POLITICO Europe
Facial-recognition ban gets lawmakers’ backing in AI Act vote.
Posted: Thu, 11 May 2023 07:00:00 GMT [source]
Once the training is finished, the system can start using predictive classification and identify objects on its own. And last but not least, the trained image recognition app should be properly tested. It will check the created model, how precise and useful it is, what its performance is, if there are any incorrect identification patterns, etc. With time the image recognition app will improve its skills and provide impeccable results. As we finish this article, we’re seeing image recognition change from an idea to something real that’s shaping our digital world.
Satellite Imagery Analysis
To begin with, let’s define image recognition and find out what’s so special about this technology. In general image recognition is a specific mechanism that is used to identify an object or subject on the given image and to perform image classification the way people can do it. In other words, image recognition is the technology that can be trained to see necessary objects. However, it can barely be called a huge novelty, since we use it now on a daily basis.
Your picture dataset feeds your Machine Learning tool—the better the quality of your data, the more accurate your model. Let’s dive deeper into the key considerations used in the image classification process. The algorithm uses an appropriate classification approach to classify observed items into predetermined classes. Now, the items you added as tags in the previous step will be recognized by the algorithm on actual pictures. In this article, we’re running you through image classification, how it works, and how you can use it to improve your business operations.
This can be done via the live camera input feature that can connect to various video platforms via API. The outgoing signal consists of messages or coordinates generated on the basis of the image recognition model that can then be used to control other software systems, robotics or even traffic lights. Once an image recognition system has been trained, it can be fed new images and videos, which are then compared to the original training dataset in order to make predictions. This is what allows it to assign a particular classification to an image, or indicate whether a specific element is present.
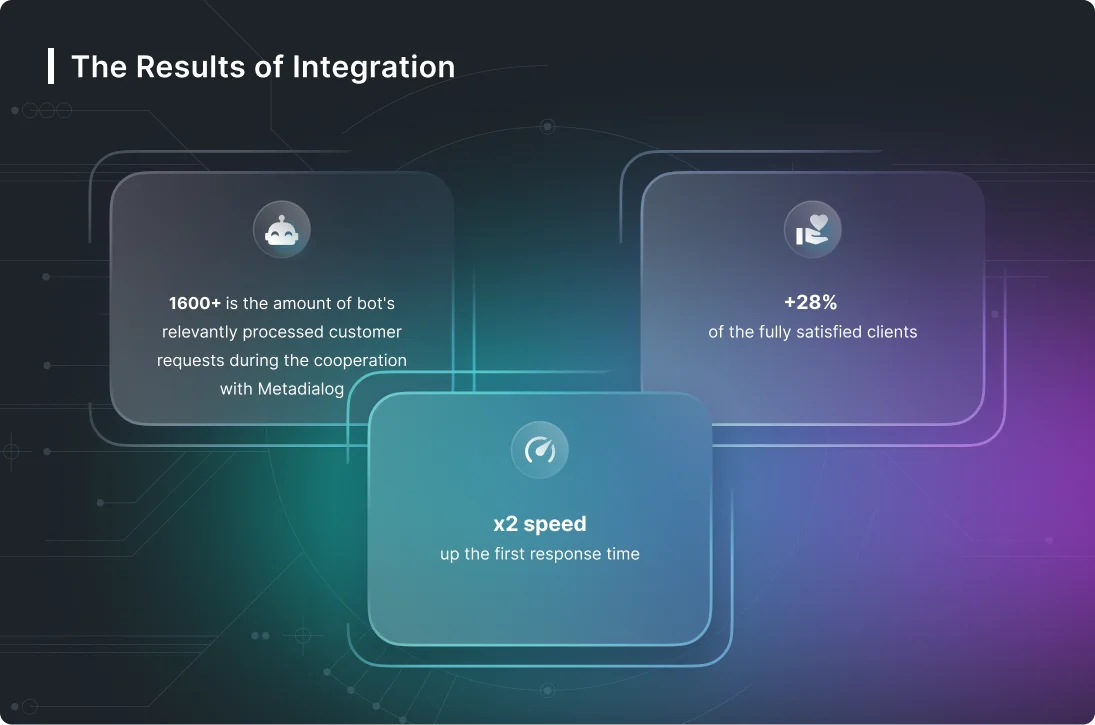
In certain cases, it’s clear that some level of intuitive deduction can lead a person to a neural network architecture that accomplishes a specific goal. Despite being 50 to 500X smaller than AlexNet (depending on the level of compression), SqueezeNet achieves similar levels of accuracy as AlexNet. This feat is possible thanks to a combination of residual-like layer blocks and careful attention to the size and shape of convolutions. SqueezeNet is a great choice for anyone training a model with limited compute resources or for deployment on embedded or edge devices. Multiclass models typically output a confidence score for each possible class, describing the probability that the image belongs to that class.
What is Image Recognition and How it is Used?
In addition to this, future use cases include authentication purposes – such as letting employees into restricted areas – as well as tracking inventory or issuing alerts when certain people enter or leave premises. But it is business that is unlocking the true potential of image processing. According to Statista, Facebook and Instagram users alone add over 300,000 images to these platforms each minute. In today’s world, where data can be a business’s most valuable asset, the information in images cannot be ignored.
Most image recognition apps are built using Python programming language and are powered up by machine learning and artificial intelligence. We decided to cover the tech part in detail, so that you can fully delve into this topic. Some people still think that computer vision and image recognition are the same thing. Computer vision models are generally more complex because they detect objects and react to them not only in images, but videos & live streams as well. A computer vision model is generally a combination of techniques like image recognition, deep learning, pattern recognition, semantic segmentation, and more.
This has led to a coarse, yet convoluted, description of how natural vision systems operate in order to solve certain vision-related tasks. These results have led to a sub-field within computer vision where artificial systems are designed to mimic the processing and behavior of biological systems at different levels of complexity. Also, some of the learning-based methods developed within computer vision (e.g. neural net and deep learning based image and feature analysis and classification) have their background in neurobiology. The Neocognitron, a neural network developed in the 1970s by Kunihiko Fukushima, is an early example of computer vision taking direct inspiration from neurobiology, specifically the primary visual cortex.
The combination of modern machine learning and computer vision has now made it possible to recognize many everyday objects, human faces, handwritten text in images, etc. We’ll continue noticing how more and more industries and organizations implement image recognition and other computer vision tasks to optimize operations and offer more value to their customers. Other machine learning algorithms include Fast RCNN (Faster Region-Based CNN) which is a region-based feature extraction model—one of the best performing models in the family of CNN. Single-shot detectors divide the image into a default number of bounding boxes in the form of a grid over different aspect ratios.
Deep image and video analysis have become a permanent fixture in public safety management and police work. AI-enabled image recognition systems give users a huge advantage, as they are able to recognize and track people and objects with precision across hours of footage, or even in real time. Solutions of this kind are optimized to handle shaky, blurry, or otherwise problematic images without compromising recognition accuracy.
The network, called the Neocognitron, included convolutional layers in a neural network. Computer vision trains machines to perform these functions, but it has to do it in much less time with cameras, data and algorithms rather than retinas, optic nerves and a visual cortex. Because a system trained to inspect products or watch a production asset can analyze thousands of products or processes a minute, noticing imperceptible defects or issues, it can quickly surpass human capabilities. Neural networks are a type of machine learning modeled after the human brain. Here’s a cool video that explains what neural networks are and how they work in more depth. Computer vision is a set of techniques that enable computers to identify important information from images, videos, or other visual inputs and take automated actions based on it.
- We therefore only need to feed the batch of training data to the model.
- Once each image is converted to thousands of features, with the known labels of the images we can use them to train a model.
- Predominant among them is the need to understand how the underlying technologies work, and the safety and ethical considerations required to guide their use.
- Image recognition can be actively used to perform medical image analysis.
- The dataset provides all the information necessary for the AI behind image recognition to understand the data it “sees” in images.
- Yet, they can be trained to interpret visual information using computer vision applications and image recognition technology.
Read more about https://www.metadialog.com/ here.